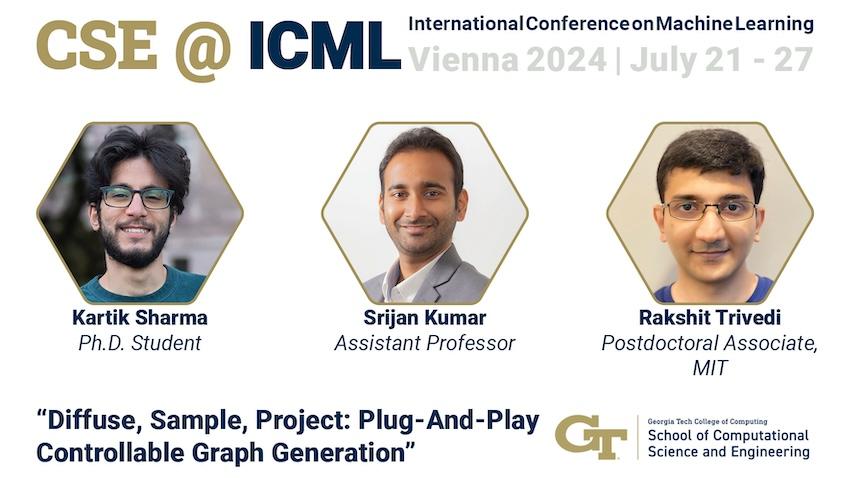
New Machine Learning Method Lets Scientists Use Generative AI to Design Custom Molecules and Other Complex Structures
New research from Georgia Tech is giving scientists more control options over generative artificial intelligence (AI) models in their studies. Greater customization from this research can lead to discovery of new drugs, materials, and other applications tailor-made for consumers.
The Tech group dubbed its method PRODIGY (PROjected DIffusion for controlled Graph Generation). PRODIGY enables diffusion models to generate 3D images of complex structures, such as molecules from chemical formulas.
Scientists in pharmacology, materials science, social network analysis, and other fields can use PRODIGY to simulate large-scale networks. By generating 3D molecules from multiple graph datasets, the group proved that PRODIGY could handle complex structures.
In keeping with its name, PRODIGY is the first plug-and-play machine learning (ML) approach to controllable graph generation in diffusion models. This method overcomes a known limitation inhibiting diffusion models from broad use in science and engineering.
“We hope PRODIGY enables drug designers and scientists to generate structures that meet their precise needs,” said Kartik Sharma, lead researcher on the project. “It should also inspire future innovations to precisely control modern generative models across domains.”
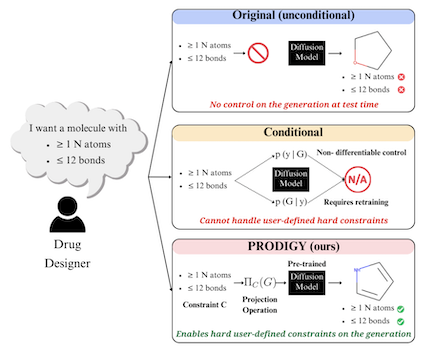
PRODIGY works on diffusion models, a generative AI model for computer vision tasks. While suitable for image creation and denoising, diffusion methods are limited because they cannot accurately generate graph representations of custom parameters a user provides.
PRODIGY empowers any pre-trained diffusion model for graph generation to produce graphs that meet specific, user-given constraints. This capability means, as an example, that a drug designer could use any diffusion model to design a molecule with a specific number of atoms and bonds.
The group tested PRODIGY on two molecular and five generic datasets to generate custom 2D and 3D structures. This approach ensured the method could create such complex structures, accounting for the atoms, bonds, structures, and other properties at play in molecules.
Molecular generation experiments with PRODIGY directly impact chemistry, biology, pharmacology, materials science, and other fields. The researchers say PRODIGY has potential in other fields using large networks and datasets, such as social sciences and telecommunications.
These features led to PRODIGY’s acceptance for presentation at the upcoming International Conference on Machine Learning (ICML 2024). ICML 2024 is the leading international academic conference on ML. The conference is taking place July 21-27 in Vienna.
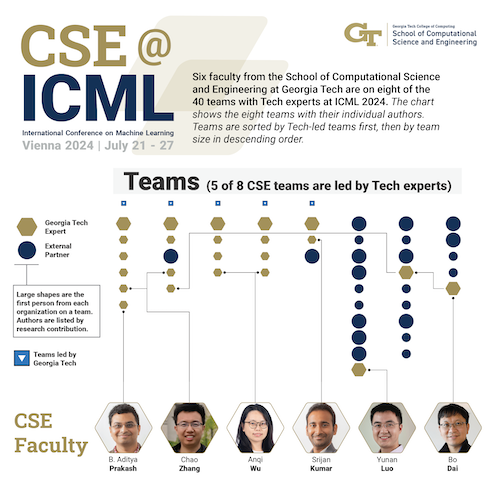
Assistant Professor Srijan Kumar is Sharma’s advisor and paper co-author. They worked with Tech alumnus Rakshit Trivedi (Ph.D. CS 2020), a Massachusetts Institute of Technology postdoctoral associate.
Twenty-four Georgia Tech faculty from the Colleges of Computing and Engineering will present 40 papers at ICML 2024. Kumar is one of six faculty representing the School of Computational Science and Engineering (CSE) at the conference.
Sharma is a fourth-year Ph.D. student studying computer science. He researches ML models for structured data that are reliable and easily controlled by users. While preparing for ICML, Sharma has been interning this summer at Microsoft Research in the Research for Industry lab.
“ICML is the pioneering conference for machine learning,” said Kumar. “A strong presence at ICML from Georgia Tech illustrates the ground-breaking research conducted by our students and faculty, including those in my research group.”
Visit https://sites.gatech.edu/research/icml-2024 for news and coverage of Georgia Tech research presented at ICML 2024.
As computing revolutionizes research in science and engineering disciplines and drives industry innovation, Georgia Tech leads the way, ranking as a top-tier destination for undergraduate computer science (CS) education. Read more about the college's commitment:… https://t.co/9e5udNwuuD pic.twitter.com/MZ6KU9gpF3
— Georgia Tech Computing (@gtcomputing) September 24, 2024