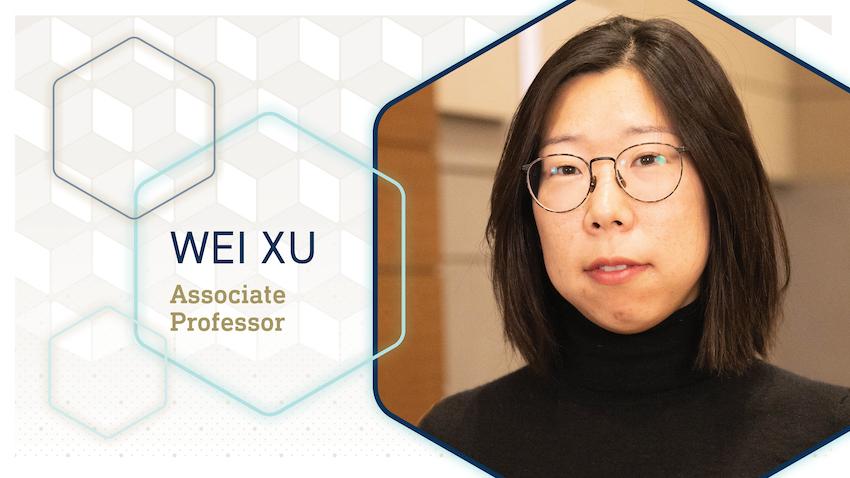
LLMs Generate Western Bias Even When Trained with Non-Western Languages
Large language models tend to exhibit Western cultural bias even when they are prompted by or trained on non-English languages like Arabic, Georgia Tech researchers have learned.
A new paper authored by researchers in Georgia Tech's School of Interactive Computing reveals these models have trouble understanding contextual nuances that are specific to non-Western cultures.
Ph.D. student Tarek Naous and his advisors, associate professors Wei Xu and Alan Ritter, challenged ChatGPT-4 and an Arabic-specific LLM to choose the most appropriate word to complete a sentence. Some of the words it could choose from were contextually correct and would make sense within Arabic culture, while others fell within Western paradigms.
In questions asking for suggestions for food dishes, drinks, or names of Arabic women, the models chose Western responses — ravioli for food, whiskey for drinks, and Roseanne for names.
The implication is that LLMs appear to fall short in their ability to assist users who have non-Western backgrounds.
As a method of measuring cultural bias, the team also introduced CAMeL (Cultural Appropriateness Measure Set for LMs). CAMeL is a benchmark data set that includes 628 naturally occurring prompts and 20,368 entities spanning eight categories that contrast Arab and Western cultures.
Since the researchers announced their paper, it has received attention on social media and in external media.
To learn more about the authors and their work, read the article spotlighting them on VentureBeat.
We are thrilled to announce Vivek Sarkar as the new Dean of the College of Computing at Georgia Tech! With a distinguished career spanning academia and industry, Sarkar's leadership promises to elevate our community to new heights. https://t.co/2mX5D46cJz pic.twitter.com/LxpLTCXWZV
— Georgia Tech Computing (@gtcomputing) April 12, 2024
@GeorgiaTech's dedication to excellence in computer science (CS) has been recognized once again, with the latest U.S. News and World Report rankings unveiling the institution at 7th place overall for graduate CS studies.https://t.co/qavNUSTb7n pic.twitter.com/BcGyGBQld8
— Georgia Tech Computing (@gtcomputing) April 10, 2024