
Hybrid Machine Learning Model Untangles Web of Communication in the Brain
A new machine learning (ML) model created at Georgia Tech is helping neuroscientists better understand communications between brain regions. Insights from the model could lead to personalized medicine, better brain-computer interfaces, and advances in neurotechnology.
The Georgia Tech group combined two current ML methods into their hybrid model called MRM-GP (Multi-Region Markovian Gaussian Process).
Neuroscientists who use MRM-GP learn more about communications and interactions within the brain. This in turn improves understanding of brain functions and disorders.
“Clinically, MRM-GP could enhance diagnostic tools and treatment monitoring by identifying and analyzing neural activity patterns linked to various brain disorders,” said Weihan Li, the study’s lead researcher.
“Neuroscientists can leverage MRM-GP for its robust modeling capabilities and efficiency in handling large-scale brain data.”

MRM-GP reveals where and how communication travels across brain regions.
The group tested MRM-GP using spike trains and local field potential recordings, two kinds of measurements of brain activity. These tests produced representations that illustrated directional flow of communication among brain regions.
Experiments also disentangled brainwaves, called oscillatory interactions, into organized frequency bands. MRM-GP’s hybrid configuration allows it to model frequencies and phase delays within the latent space of neural recordings.
MRM-GP combines the strengths of two existing methods: the Gaussian process (GP) and linear dynamical systems (LDS). The researchers say that MRM-GP is essentially an LDS that mirrors a GP.
LDS is a computationally efficient and cost-effective method, but it lacks the power to produce representations of the brain. GP-based approaches boost LDS's power, facilitating the discovery of variables in frequency bands and communication directions in the brain.
Converting GP outputs into an LDS is a difficult task in ML. The group overcame this challenge by instilling separability in the model’s multi-region kernel. Separability establishes a connection between the kernel and LDS while modeling communication between brain regions.
Through this approach, MRM-GP overcomes two challenges facing both neuroscience and ML fields. The model helps solve the mystery of intraregional brain communication. It does so by bridging a gap between GP and LDS, a feat not previously accomplished in ML.
“The introduction of MRM-GP provides a useful tool to model and understand complex brain region communications,” said Li, a Ph.D. student in the School of Computational Science and Engineering (CSE).
“This marks a significant advancement in both neuroscience and machine learning.”
Fellow doctoral students Chengrui Li and Yule Wang co-authored the paper with Li. School of CSE Assistant Professor Anqi Wu advises the group.
Each MRM-GP student pursues a different Ph.D. degree offered by the School of CSE. W. Li studies computer science, C. Li studies computational science and engineering, and Wang studies machine learning. The school also offers Ph.D. degrees in bioinformatics and bioengineering.
Wu is a 2023 recipient of the Sloan Research Fellowship for neuroscience research. Her work straddles two of the School’s five research areas: machine learning and computational bioscience.
MRM-GP will be featured at the world’s top conference on ML and artificial intelligence. The group will share their work at the International Conference on Machine Learning (ICML 2024), which will be held July 21-27 in Vienna.
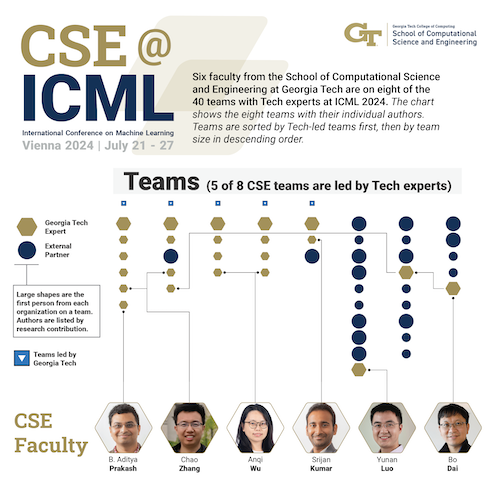
ICML 2024 also accepted for presentation a second paper from Wu’s group intersecting neuroscience and ML. The same authors will present A Differentiable Partially Observable Generalized Linear Model with Forward-Backward Message Passing.
Twenty-four Georgia Tech faculty from the Colleges of Computing and Engineering will present 40 papers at ICML 2024. Wu is one of six faculty representing the School of CSE who will present eight total papers.
The group’s ICML 2024 presentations exemplify Georgia Tech’s focus on neuroscience research as a strategic initiative.
Wu is an affiliated faculty member with the Neuro Next Initiative, a new interdisciplinary program at Georgia Tech that will lead research in neuroscience, neurotechnology, and society. The University System of Georgia Board of Regents recently approved a new neuroscience and neurotechnology Ph.D. program at Georgia Tech.
“Presenting papers at international conferences like ICML is crucial for our group to gain recognition and visibility, facilitates networking with other researchers and industry professionals, and offers valuable feedback for improving our work,” Wu said.
“It allows us to share our findings, stay updated on the latest developments in the field, and enhance our professional development and public speaking skills.”
Visit https://sites.gatech.edu/research/icml-2024 for news and coverage of Georgia Tech research presented at ICML 2024.
As computing revolutionizes research in science and engineering disciplines and drives industry innovation, Georgia Tech leads the way, ranking as a top-tier destination for undergraduate computer science (CS) education. Read more about the college's commitment:… https://t.co/9e5udNwuuD pic.twitter.com/MZ6KU9gpF3
— Georgia Tech Computing (@gtcomputing) September 24, 2024