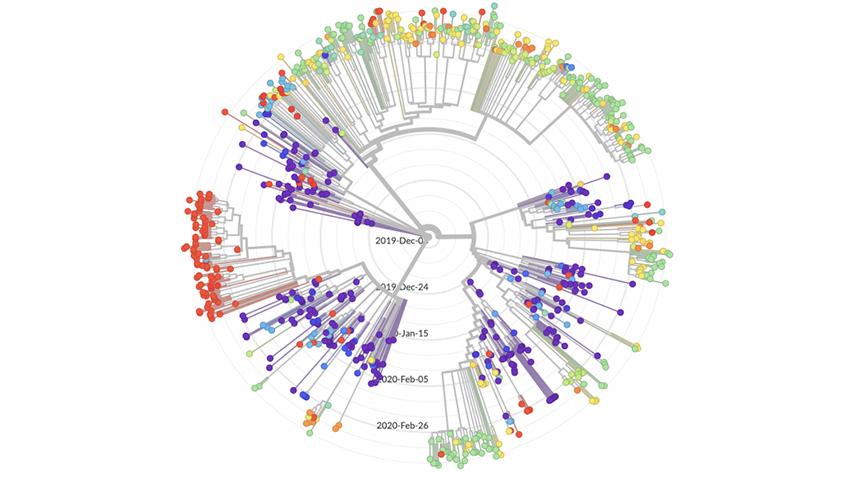
Scientists Collaborating on New Data-Driven Approach to Covid-19 Intervention
As the number of Covid-19 cases rise in the United States and businesses reopen, health officials report that it is becoming increasingly difficult to conduct effective contact tracing and quarantine appropriately.
To help these officials, Georgia Tech researchers are collaborating with peers from the University of Virginia (UVA) to improve Covid-19 surveillance by designing more targeted and adaptive testing and intervention techniques.
Led by Georgia Tech School of Computational Science and Engineering Associate Professor B. Aditya Prakash, the team is developing these techniques using a new data-driven approach that brings together different types of datasets.
According to Prakash, standard preventative measures are proving to be substantially harder for scientists and public health experts to implement as infection rates continue to rise in many parts of the country. This is complicated further by factors such as asymptomatic transmission, high incubation periods, human mobility, weather patterns, and limited testing.
“The basic motivation [of our research] is that as the number of coronavirus cases rise, it becomes harder to target, quarantine, and prevent the spread of the disease,” said Prakash.
“To improve Covid-19 surveillance abilities in a data-driven fashion, we plan to augment and align different types of datasets. This will help us adaptively understand which new infections are more likely high risk, where we should focus our attentions on quarantine efforts, and also better model and understand the disease spread.”
Bolstered by a National Science Foundation (NSF) Rapid Response Research (RAPID) grant, this project will integrate several datasets including line lists, which show case history, and other auxiliary data sets, such as census records, mobility and strain data. The project is attempting an ambitious approach that aims to bridge these widely contrasting data sets to create actionable insights.
Using these datasets, the researchers will computationally infer transmission chains to new infections.
“Our prior works on interventions and surveillance have been highly successful in this regard. Our inferred chains naturally give us guidance on whom to adaptively surveil and quarantine among the new infections,” said Prakash.
“We believe that the current patient line lists have a lot of missing information. This can be true for many diseases, but especially so in the case of Covid-19 where asymptomatic transmission seems to play such a large role in its spread,” he said.
“We are developing a sort of risk measure to understand where these missing clusters may be, such as near someone who is a highly mobile person living in a heavily populated area.”
Prakash and his UVA collaborators, including Biocomplexity Institute Professor Anil Vullikanti, have been working on epidemiological models for several years and have developed their own data sets for different geographical areas. As a proof of concept, their team is first focusing this research on the geographical regions of Georgia and Virginia and plans to extend later to other regions nationwide.
Apart from the immediate applications to the Covid-19 pandemic, the tools developed through this research will be broadly applicable to other infectious disease settings such as influenza.
The project will release its methods, which can be used by both practitioners and modelers for faster surveillance and testing under resource constraints.
For more coverage of Georgia Tech’s response to the coronavirus pandemic, please visit our Responding to COVID-19 page.
[Related Link: Georgia Tech Professor Leads Multi-Institution Team in Combatting Hospital Acquired Infections]
[Related Link: Team Using Deep Learning to Forecast Pandemic in the U.S.]
As computing revolutionizes research in science and engineering disciplines and drives industry innovation, Georgia Tech leads the way, ranking as a top-tier destination for undergraduate computer science (CS) education. Read more about the college's commitment:… https://t.co/9e5udNwuuD pic.twitter.com/MZ6KU9gpF3
— Georgia Tech Computing (@gtcomputing) September 24, 2024