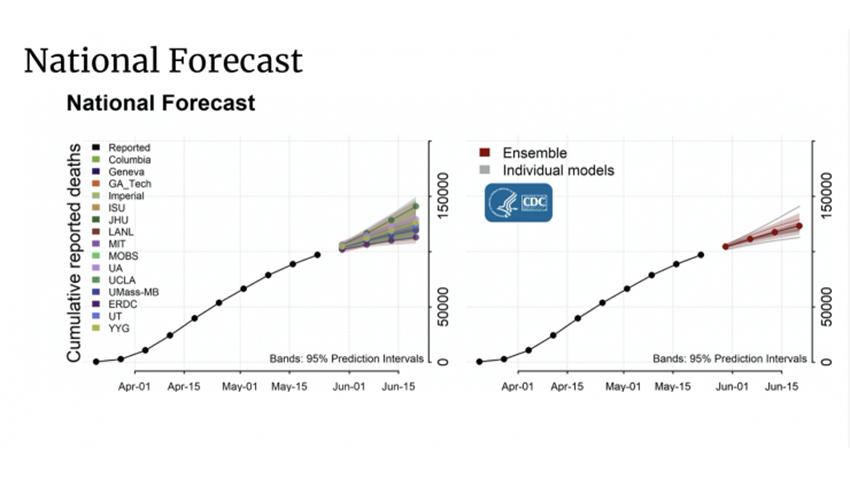
Team Using Deep Learning to Forecast Pandemic in the U.S.
The Centers of Disease Control and Prevention (CDC) is hosting forecasting projects to predict the Covid-19 spread, number of hospitalizations, flu-like-symptoms, and deaths caused by the disease across the country.
This critically timed effort is comprised of a handful of teams which include data scientists, epidemiologists, statisticians, and high-performance computing (HPC) researchers from national laboratories, public universities, public health institutions, and some private sector agents.
Georgia Tech’s School of Computational Science and Engineering (CSE) Associate Professor B. Aditya Prakash and CSE Ph.D. student Alexander Rodriguez are leading one of the collaborative teams on these projects and are using a new data-driven approach to disease forecasting.
Their team is using deep learning models to forecast specific targets related to the trajectory of Covid-19 at the national, regional, state, and local levels. The CDC synthesizes their weekly and monthly predictions with other models to help determine policy and other planning decisions to help communities prepare for and fight the disease.
“We want to predict early to give lead times to decision makers to decide appropriately when to and how to allocate resources such as determining where to send ventilators, where additional beds are most critically needed, vaccine creation timelines, implementing temporary shelter in-place orders, whether additional guidance to state and local authorities is needed, and more,” said Prakash.
Prakash is an expert in using data science for epidemiology and infectious diseases and has been a lead team researcher on preexisting influenza forecasting projects with the CDC since 2018. He is also part of a recently awarded National Science Foundation (NSF) Expeditions in Computational Epidemiology grant that is actively working with multiple federal and state agencies to support response efforts for the current pandemic. Prakash’s portion of the project is aimed toward developing data science methods for public health problems ranging from epidemic detection to inference and control. He will also use Georgia Tech’s largest HPC resource, the Hive supercomputer, for running his large-scale models.
The Aid of a Public Health Surveillance Network
By using data captured by the U.S. Outpatient Influenza-like Illness Surveillance Network (ILINet), and the Covid-19 Associated Surveillance Network, the forecasting teams are provided with real-time health data from health providers across the United States.
“Predicting when diseases will peak, when they will be above a certain baseline, when they will be below a baseline, and so on, is all very useful,” said Prakash. “The CDC has run similar influenza forecasting projects for the past few years in which all the teams taking part in the challenge forecast real-time predictions for flu-like illness across the US.”
“So, after Covid-19 started, naturally one big question that arose was, ‘Can we use this type of system to do Covid-19 forecasting?’,” he said.
After a few months of planning and working with various stakeholders like local and national health partners, and in consultation with the forecasting teams, the CDC set up multiple Covid forecasting projects, each predicting different metrics related to the trajectory.
“It was great to see these groups come together quickly,” said Prakash.
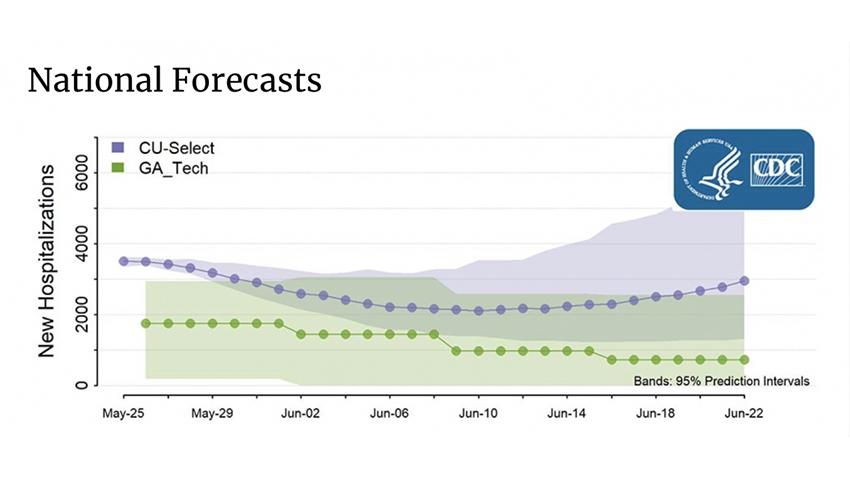
Current Benefits and Challenges Facing Forecasting Models
In addition to CDC ILI and Covid data, Prakash’s team is incorporating many other real-time datasets such as syndromic surveillance data and point-of-care data from leading providers. His team combines these datasets with domain knowledge using end-to-end deep learning models to predict targets on a weekly basis. Currently, their team - which includes University of Illinois at Urbana-Champaign Professor Jimeng Sun, Danica Xiao and Cheng Qiang of IQVIA, and Virginia Tech Professor Naren Ramakrishnan - is focusing more on near-term forecasts as opposed to very long-term projections.
However, according to Prakash, there are added challenges in tracking and predicting the Covid-19 disease spread for both traditional models and his team’s new deep learning model. This includes the fact that people are still learning about the epidemiology of the virus – such as the proportion of asymptomatic cases, new data surrounding the epidemic is being continuously added – such as new tests, and there is a large heterogeneity in the evolving US response – such as the wide variety of interventions and strategies implemented across the different states.
Another added challenge is that there is no real historical data to compare to, as it is a novel virus. For instance, Prakash’s team’s historical model was based on past influenza seasons. This will become even more challenging in the fall season when any Covid-19 cases will coincide with the usual flu season in the US.
Due to these added factors, Prakash’s team has to diligently avoid pitfalls of blanket assumptions from the model – an issue which they are addressing by adding an increased layer of interpretability for their model’s output. They hope that the forecasts help the decision makers see what they should expect in the near future.
“The coronavirus pandemic is like a natural disaster, if you can predict it earlier then that is better, as you want longer lead times to prepare. However, this is unlike weather forecasting, which also aims to help communities prepare. Human decisions do not change whether it is going to be sunny or not,” said Prakash.
“In contrast, human behavior can change the outcome of an epidemic. If we predict it’s going to be large outbreak, and everyone decides to stay home, it will fizzle out and nullify the prediction, and that’s a good thing! This is the tricky part of disease forecasting.”
Currently, one of the biggest challenges the forecasting teams face, stresses Prakash, is quickly detecting, predicting, and reacting if any secondary waves of infection begin.
For more coverage of Georgia Tech’s response to the coronavirus pandemic, please visit our Responding to COVID-19 page.
We are thrilled to announce Vivek Sarkar as the new Dean of the College of Computing at Georgia Tech! With a distinguished career spanning academia and industry, Sarkar's leadership promises to elevate our community to new heights. https://t.co/2mX5D46cJz pic.twitter.com/LxpLTCXWZV
— Georgia Tech Computing (@gtcomputing) April 12, 2024
@GeorgiaTech's dedication to excellence in computer science (CS) has been recognized once again, with the latest U.S. News and World Report rankings unveiling the institution at 7th place overall for graduate CS studies.https://t.co/qavNUSTb7n pic.twitter.com/BcGyGBQld8
— Georgia Tech Computing (@gtcomputing) April 10, 2024