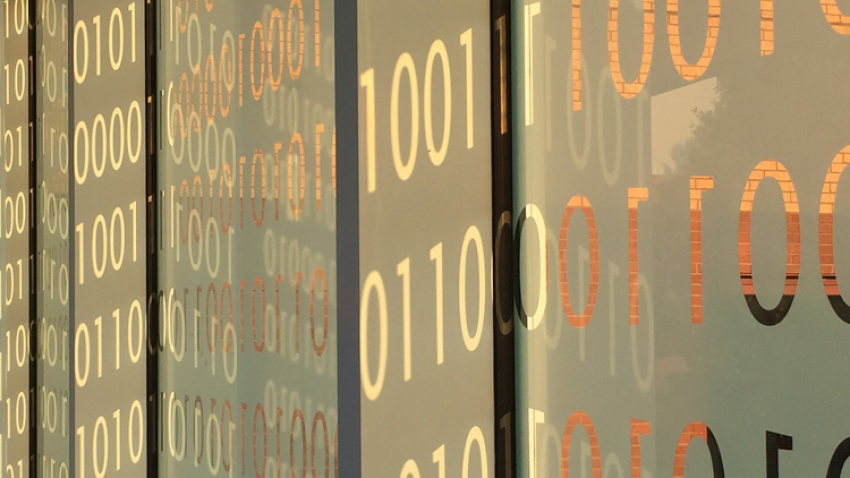
Deep Learning Model Cuts Time to Produce Hi-Res. Land Cover Maps
A new approach enables the first large-scale charting method that can quickly create high-resolution land maps at a fraction of the cost.
Identifying land cover types from high-resolution satellite imagery is crucial to addressing many sustainability-related issues. However, the process of mapping this information is expensive, computationally complex, and time-consuming.
As a result, portions of these maps can be outdated before they reach conservationists, planners, researchers, and others that rely on this information to make decisions impacting the environment. To provide these groups with timely and actionable land cover maps, a Microsoft research team led by Georgia Tech Ph.D. student Caleb Robinson has developed a new approach that combines satellite and aerial imager with advanced deep learning techniques.
The result is the first high-resolution land cover map of the contiguous United States.
“The goal is to identify and summarize changes in land cover on the scale of days,” said Robinson. “The scale of days is extremely important as this can inform conservation agencies as to how effective their interventions are or this can highlight potential problem areas such as rapid loss of forest cover.”
The new web-based mapping tool is funded by Microsoft AI for Earth and can show daily changes in land cover types such as water, forests, fields, and human developments. It uses a method originally developed for electron microscopy known as multi-resolution data fusion. The method scans all of the imagery at a low resolution, while also collecting a fraction of hi-res. data points at the same time. The two are then merged using existing modeling techniques to create high-quality maps that can be updated in a matter of days rather than in weeks or even months.
“Other deep learning–based methods for land cover mapping have shown to be effective but are limited in their scale,” said Robinson. “By developing methods for generalizing models to new regions, we have achieved high-quality results for the entire U.S.”
To highlight the effectiveness of their new approach the team points to a land cover map of the Chesapeake Bay Watershed. The Chesapeake Conservancy spent 10 months and $1.3 million to produce the map for an approximately 64,299 square-mile area. In comparison, the team using its new tool was able to produce a hi-res. land cover map of the contiguous U.S. – all 3.20 million square miles of it – within a week for a cost of $5,000.
Bistra Dilkina, a School of Computational Science and Engineering adjunct professor and researcher who specializes in technology for conservation, is a co-investigator on this project and Robinson’s advisor.
"Overall, artificial intelligence has a lot to offer in helping conserve our environment, from land cover mapping and detection of degradation to optimizing conservation planning and predicting risk of illegal wildlife poaching in protected areas,” she said.
The deep learning method and a detailed account of how the team collaborated with the Chesapeake Conservancy is outlined in a paper, presented at the premier annual computer vision conference, CVPR 2019, on June 20.
An addition to the map outlined in the paper is a new web interface that allows users to:
- Explore land cover model predictions
- Fine-tune the model to new geographic areas
- Extend the model with new, user-defined classes
- Download resulting land cover data
The code for training and testing these models can be accessed here.
As computing revolutionizes research in science and engineering disciplines and drives industry innovation, Georgia Tech leads the way, ranking as a top-tier destination for undergraduate computer science (CS) education. Read more about the college's commitment:… https://t.co/9e5udNwuuD pic.twitter.com/MZ6KU9gpF3
— Georgia Tech Computing (@gtcomputing) September 24, 2024