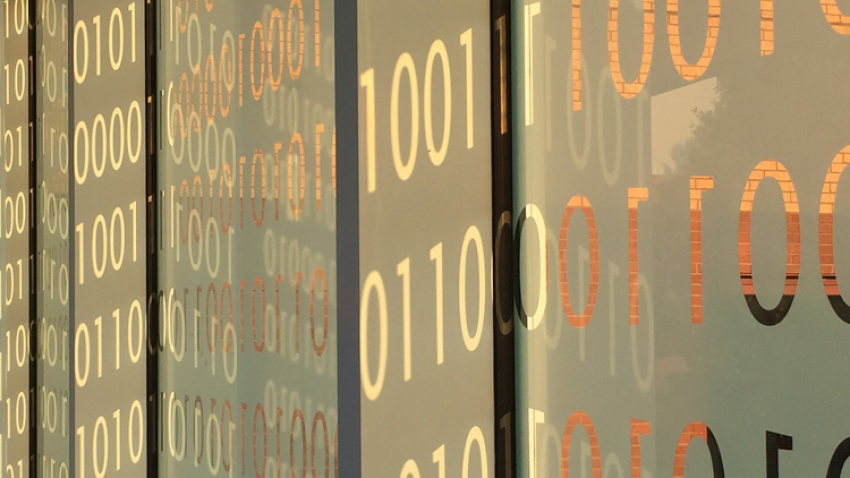
Using Data Science to Fix the Flint Water Crisis
The lead contamination in Flint’s drinking water is considered one of the biggest environmental crises of the recent years. Finding the locations of tens of thousands of lead pipes throughout the city seemed an insurmountable challenge to recovery efforts until a team of data scientists stepped in, including School of Computer Science Assistant Professor Jacob Abernethy.
What started as an attempt to save money by switching the Michigan city’s water supply from the Detroit system to the Flint River in 2014 led to contaminated water and international outcry by 2016. Lead wasn’t coming from the water itself, but the lead service lines that connected Flint homes to the city’s water system.
The problem was no one knew how many homes were affected. Flint hadn’t complied with Environmental Protection Agency standards to keep records of lead service lines. With as many as 50,000 homes potentially needing service line replacements, the city was facing costs up to $250 million. Flint officials were granted only $27 million from the state legislature and an additional $100 million from the U.S. government toward recovery efforts.
Google believed data science could expedite the process of identifying specific homes in need of replacement service lines and donated to the University of Michigan and Flint’s Community Foundation. Abernethy, then an assistant professor at the Ann Arbor campus, and his Data Science Team of students were called in.
Deciding with data
By aggregating a mix of datasets — including pipe material information, parcel records, U.S. census reports, city infrastructure maps, and water samples — Abernethy and his team took advantage of a machine learning technique known as active learning in order to determine which homes were most likely to have lead service lines.
The algorithm performed three processes simultaneously: a statistical model that predicted which portion of the service line was lead, copper, or otherwise; a decision procedure that randomly generated homes for inspection; and a decision-making algorithm that chose which homes should have lines replaced.
Using the predictive model allowed better targeting of resources. Had this method been fully utilized throughout the replacement process, the team estimates that the city could have saved over $10 million — enough money to replace pipes at 2,000 more homes, according Abernethy. The team also created a web-based mobile application to help government offices, residents, and contractors coordinate efforts and update their data in real-time.
Working with a community
Having access to all of this data, as well as numerous algorithmic tools, did not mean that all could be easily implemented on the ground in Flint. Every service line replacement had to be approved by the Flint City Council, and, according to Abernethy, university academics are not always appreciated in towns with struggling economies.
“You write these papers that have these abstract things like, ‘Here’s the way to optimize this whole procedure to save money,’ but what we proposed would take changing large bureaucratic structures and procedures,” Abernethy said. “These interventions are not always welcome.”
Yet the relationship went both ways. For most of the team, this was the first time their research had such a direct impact on a community. Abernethy recalled a city council meeting when a councilwoman asked if her home’s water pipes could be replaced next so that her grandchildren could have safe water to drink.
“It was very touching and moving to have this interaction when we had been thinking of this as an optimization problem,” Abernethy said. “It brought home what we were doing. This is great we’re helping someone like you, but this woman may not have her pipes replaced because of some line in my code. For me, personally, the stakes went up after this conversation.”
Abernethy considers his work in Flint to be one of the most rewarding and challenging of his career.
“If we had picked a project that didn’t have this much public attention, we probably would’ve just written a paper and given up and not done anything useful,” he said. “This was an issue where we had the incentives to go in and do this work very thoughtfully and deeply and really make a difference. I’m really proud of that, and feel very lucky to have had the opportunity to engage in such an important issue.”
Abernethy presents the research in the paper, Active Remediation: The Search for Lead Pipes in Flint, Michigan, coauthored with University of Michigan faculty and students including Assistant Professor of Marketing Eric Schwartz, Alex Chojnacki, Arya Farahi, and Brigham Young University Ph.D. student Jared Webb. The work won the best student paper at the Knowledge Discovery and Data Mining (KDD) conference in London from August 19-23.
As computing revolutionizes research in science and engineering disciplines and drives industry innovation, Georgia Tech leads the way, ranking as a top-tier destination for undergraduate computer science (CS) education. Read more about the college's commitment:… https://t.co/9e5udNwuuD pic.twitter.com/MZ6KU9gpF3
— Georgia Tech Computing (@gtcomputing) September 24, 2024