Upcoming Events
School of CSE Seminar Series: Youssef Marzouk
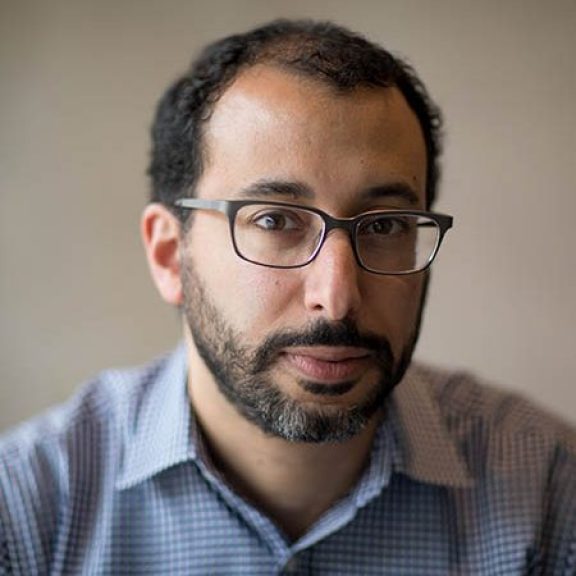
Speaker: MIT Professor Youssef Marzouk
Date and Time: April 12, 2:00-3:00 p.m.
Location: Scheller 201
Host: Peng Chen
Title: On Low-Dimensional Structure in Transport and Inference
Abstract: Transportation of measure underlies many powerful tools for Bayesian inference, density estimation, and generative modeling. The central idea is to deterministically couple a probability measure of interest with a tractable “reference” measure (e.g., a standard Gaussian). Such couplings are induced by transport maps, and enable direct simulation from the desired measure simply by evaluating the transport map at samples from the reference.
While an enormous variety of representations and constructive algorithms for transport maps have been proposed in recent years, it is inevitably advantageous to exploit the potential for low-dimensional structure in the associated probability measures. I will discuss two such notions of low-dimensional structure, and their interplay with transport-driven methods for sampling and inference. The first seeks to approximate a high-dimensional target measure as a low-dimensional update of a dominating reference measure. The second is low-rank conditional structure, where the goal is to replace conditioning variables with low-dimensional projections or summaries. In both cases, under appropriate assumptions on the reference or target measures, one can derive gradient-based upper bounds on the associated approximation error and minimize these bounds to identify good subspaces for approximation. The associated subspaces then dictate specific structural ansatzes for transport maps that represent the target of interest.
I will showcase several algorithmic instantiations of this idea, focusing on Bayesian inverse problems, data assimilation, and simulation-based inference.
Bio: Youssef Marzouk is a professor in the Department of Aeronautics and Astronautics at the Massachusetts Institute of Technology (MIT), and co-director of the Center for Computational Science and Engineering within the MIT Schwarzman College of Computing. He is also a core member of MIT's Statistics and Data Science Center and a PI in the MIT Laboratory for Information and Decision Systems (LIDS).
His research interests lie at the intersection of statistical inference, computational mathematics, and physical modeling. He develops new methodologies for uncertainty quantification, Bayesian computation, and machine learning in complex physical systems, motivated by a broad range of engineering and science applications. His recent efforts have centered on algorithms for data assimilation and inverse problems; dimension reduction methodologies for high-dimensional learning and surrogate modeling; optimal experimental design; and transportation of measure as a tool for inference and stochastic modeling.
He received his S.B., S.M., and Ph.D. degrees from MIT and spent four years at Sandia National Laboratories before joining the MIT faculty in 2009. He is also an avid coffee drinker and classical pianist.
Event Details
Media Contact
Peng Chen (pchen402@gatech.edu)
EVENTS BY SCHOOL & CENTER
School of Computational Science and Engineering
School of Interactive Computing
School of Cybersecurity and Privacy
Algorithms and Randomness Center (ARC)
Center for 21st Century Universities (C21U)
Center for Deliberate Innovation (CDI)
Center for Experimental Research in Computer Systems (CERCS)
Center for Research into Novel Computing Hierarchies (CRNCH)
Constellations Center for Equity in Computing
Institute for People and Technology (IPAT)
Institute for Robotics and Intelligent Machines (IRIM)