Upcoming Events
ML Virtual Seminar: Learning Locomotion: From Simulation to Real World
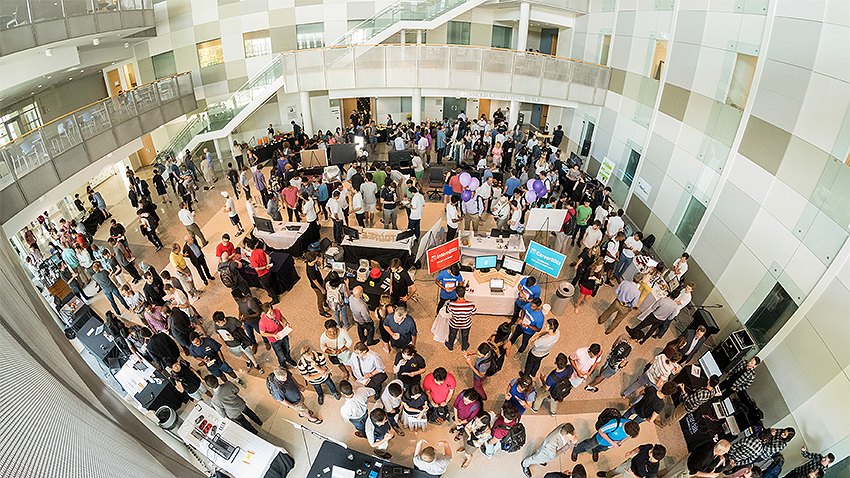
Abstract: Deep Reinforcement Learning (DRL) holds the promise of designing complex robotic controllers automatically. In this talk, I will discuss two different approaches to apply deep reinforcement learning to learn locomotion controllers for legged robots. The first approach is through sim-to-real transfer. Due to safety concerns and limited data, most of the training is conducted in simulation. However, controllers learned in simulation usually perform poorly on real robots. I will present a set of techniques to overcome this sim-to-real gap. The second approach is to directly learn in the real world. Due to the complexity and diversity of the real environments, building a simulation that can faithfully model the real world is not always feasible. Having the ability to learn on the fly and adapt quickly in real-world scenarios is crucial for large-scale deployment of robots. I will discuss the challenges of training legged robots in the real world and various ways to address these challenges.
Bio: Tan's research is to develop safe machine learning techniques and apply them to real-world systems, such as robots and power grids. His research enables robots to automatically learn skills to accomplish complex tasks in the real world. His research interests include AI safety, machine learning, robotics, power grids and simulation. He is currently the Tech Lead Manager of the Robot Locomotion and Safety teams at Google Brain Robotics. The mission of the team is to build safe, autonomous and agile mobile robots that can work seamlessly in human-centered environments. Tan earned his PhD at the Computer Graphics Laboratory at Georgia Tech, advised by Greg Turk and Karen Liu.
Register and Attend: https://primetime.bluejeans.com/a2m/register/rhvgtpaa
Event Details
Media Contact
Kyla Hanson
EVENTS BY SCHOOL & CENTER
School of Computational Science and Engineering
School of Interactive Computing
School of Cybersecurity and Privacy
School of Computing Instruction
Algorithms and Randomness Center (ARC)
Center for 21st Century Universities (C21U)
Center for Deliberate Innovation (CDI)
Center for Experimental Research in Computer Systems (CERCS)
Center for Research into Novel Computing Hierarchies (CRNCH)
Constellations Center for Equity in Computing
Institute for People and Technology (IPAT)
Institute for Robotics and Intelligent Machines (IRIM)